AI in recruitment: garbage in, garbage out
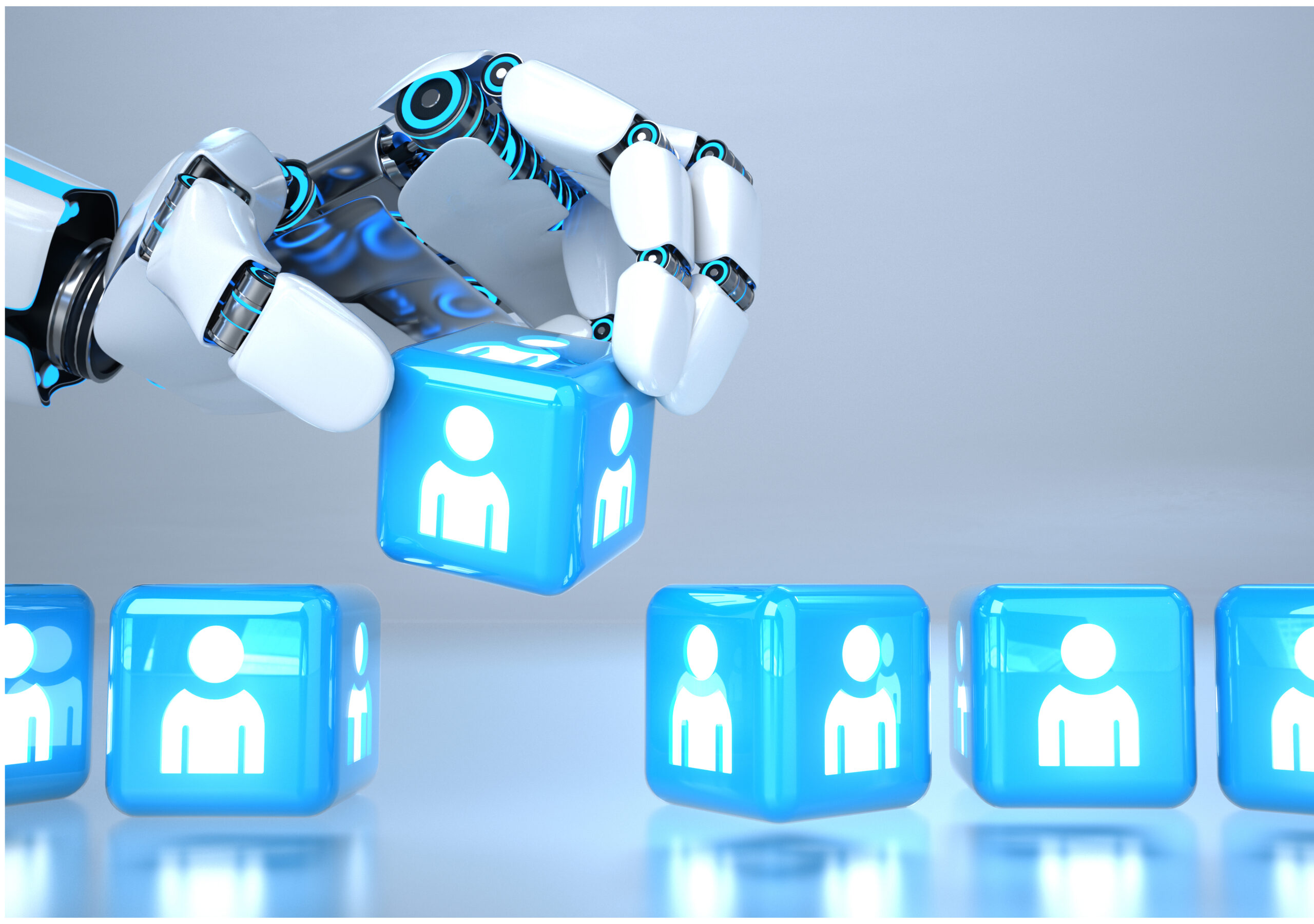
AI is an incredibly powerful tool with many applications; but questions need to be asked about its usage in the recruitment sector.
We wrote about AI recruitment technology a few years ago, and the picture hasn’t changed much, except in its uptake: AI recruitment tools now proliferate.
For people who have entered the job market in the last few years, HireVue has become a dreaded part of application processes. Even pre-pandemic, competitive grad schemes relied on HireVue as a first stage filter to their applicant pool, minimizing employee time used, while maximizing applications slashed. The reasoning behind this is fair enough: Goldman Sachs receives 250 000 applications for their graduate positions, and the rate of offers made for the big players in professional services are famously in single digits.
No surprise, though, that this shortcut has drawbacks. A Cornell University study titled “Image Representations Learned with Unsupervised Pre-Training Contain Human-like Biases,” found that the machine-learning models that AI tools used by products like HireVue are based on large datasets sourced from the internet for cost-saving purposes. The conclusion of the survey: “Our results suggest that unsupervised image models learn human biases from the way people are portrayed in images on the web. These findings serve as a caution for computer vision practitioners using transfer learning: pre-trained models may embed all types of harmful human biases from the way people are portrayed in training data, and model design choices determine whether and how those biases are propagated into harms downstream.” Essentially, AI technologies see images of you how the internet does. For those of us who don’t read as white men, that translates to: garbage in, garbage out.
In addition to tests of enthusiasm, willingness to learn, competencies and behaviours, and personal stability, HireVue allows current workers at a company to go through the same tests to provide a benchmark value. “The best candidates, in other words, end up looking and sounding like the employees who had done well before the prospective hires had even applied.” As we’ve reported before, cognitive diversity is a major asset to any company in encouraging innovation and problem solving. While this feature is also just replicating human biases (for solutions on how to reduce bias in your recruitment process, see our blog on the topic), we should be striving for the impartiality that technology so often claims to provide, and with it, the diversity in thought, gender, and ethnicity that invariably improves a workplace’s culture, and range of competencies.
The most shocking example of this came a few years ago from Amazon, when it was revealed that their in-house computer programme to review CVs penalised any mention of women. “That is because Amazon’s computer models were trained to vet applicants by observing patterns in resumes submitted to the company over a 10-year period. Most came from men, a reflection of male dominance across the tech industry.” Amazon duly ditched the programme.
An investigation by Elisa Harlan and Oliver Schnuck at Bayerischen Rundfunk (BR) into Munich-based AI start-up Retorio lays bare the problem. Like HireVue, Retorio claims to make recruitment faster and fairer. In fact, BR discovered, the algorithm paid more attention to factors like having a picture or bookcase behind you (which apparently makes you more open, conscientious and agreeable, and much less neurotic, than people with plain backgrounds), or your lighting, than what the interviewer actually said. Bizarrely, this product seems to have been deliberately designed to replicate the first impressions of interviewers. As Uwe Kanning, Professor of Business Psychology from the University of Osnabrück, commented “The software should actually be able to filter out this information in order to be better than the gut feeling of any person who is susceptible to such influences.” Retorio, for one, is actually designed to confirm the bias rather than reduce it.
Since our last report on AI in recruitment, the major change has been in the level of supervision of computer learning. OpenAI’s iGPT and Google’s SimCLR both share unsupervised learning algorithms. Older algorithms depended on images that had been labelled manually. In 2019 a study found misogynistic and racist language deeply entrenched in ImageNet, a database of manually-labelled images and the main source for training computer-vision models.
When Microsoft launched its AI-run Twitter account, TayTweets, it took mere days before it was spouting racist language and conspiracy theories. As Popular Mechanics reported, “If you take an AI and then don’t immediately introduce it to a whole bunch of trolls shouting racism at it for the cheap thrill of seeing it learn a dirty trick, you can get some more interesting results. Endearing ones even! Multiple neural networks designed to predict text in emails and text messages have an overwhelming proclivity for saying “I love you” constantly.”
The truth is that AI is phenomenally helpful in certain tasks, and it may be that, in time, it will have its uses in recruitment. But, for now, it’s worth asking whether we should integrate such developing technology into important decision-making processes that can affect lives and businesses. Our suggestion would be to hold fire on adopting AI technology as judge and jury, until we can filter out the garbage going into algorithms.
Martin Tripp Associates is a London-based executive search consultancy. While we are best-known for our work across the media, information, technology, communications and entertainment sectors, we have also worked with some of the world’s biggest brands on challenging senior positions. Feel free to contact us to discuss any of the issues raised in this blog.